What can Healthcare AI learn from Weather AI?
- Lloyd Price
- May 6, 2024
- 7 min read

Exec Summary:
AI has revolutionised the weather industry by providing faster, more accurate, and personalised weather predictions. This has significant benefits for public safety, disaster preparedness, and various industries that rely on weather information.
The widespread adoption of AI in weather forecasting is a recent phenomenon, its roots go back further than you might think:
Early applications (1970s): AI, in the form of complex algorithms running on supercomputers, has been used in weather prediction since the 1970s. These algorithms helped analyse vast amounts of data and improve forecast accuracy.
Rise of Machine Learning (2010s onwards): The real revolution in AI-powered weather forecasting started around the 2010s with the rise of machine learning (ML) techniques. These techniques allow models to learn from historical weather data and identify complex patterns that traditional models might miss.
Significant breakthroughs (2023): A significant milestone occurred in July 2023 with the publication of a paper demonstrating how AI weather models could outperform the industry standard (High Resolution Forecast) in terms of accuracy. This marked a major step forward in the potential of AI for weather prediction.
Therefore, while AI has been involved in weather forecasting for decades, the recent advancements in machine learning and the increasing availability of data have significantly boosted its impact and potential in recent years
Weather AI excels at processing massive amounts of data from various sources like satellites, radars, and historical records. Healthcare AI can adopt similar strategies to integrate and analyse diverse patient data, including medical records, environmental factors, genetic data, and sensor data from wearables.
Healthcare AI can learn several valuable things from weather AI:
1. Advanced Data Analysis and Forecasting:
Complex Data Integration: Weather AI excels at processing massive amounts of data from diverse sources like satellites, radar, sensor networks, and social media. This can inspire healthcare AI to integrate various patient data (medical records, wearables, environmental factors) for more comprehensive analysis and prediction.
Predictive Modelling: Weather AI utilises sophisticated algorithms (e.g., Random Forests, Support Vector Machines) to forecast weather patterns with high accuracy. Healthcare AI can leverage these techniques to predict disease outbreaks, individual patient health risks, and potential complications.
2. Personalisation and Individualised Care:
Tailored Interventions: Weather forecasts are personalized based on location and individual needs. Similarly, healthcare AI can personalise treatment plans and preventative measures based on a patient's medical history, genetics, and environmental factors.
Early Warning Systems: Weather AI can trigger alerts for extreme weather events. Healthcare AI can adapt this approach to provide early warnings of potential health risks or disease flare-ups based on individual patient data and environmental conditions.
3. Continuous Learning and Adaptation:
Real-time Data Processing: Weather AI constantly processes new data to update forecasts in real-time. Healthcare AI can learn to continuously analyse real-time patient data (sensor readings, medication adherence) to adjust treatment plans and interventions as needed.
Evolving Algorithms: Weather AI models are constantly being improved with new data and techniques. Healthcare AI can adopt a similar approach to continuously refine its algorithms based on new medical discoveries, treatment advancements, and patient data.
Here are some additional points to consider:
Identifying Environmental Triggers: Weather AI can help pinpoint how weather patterns (temperature, humidity, pollen count) influence specific health conditions like asthma or allergies. This can inform healthcare AI in developing preventative measures and personalised care plans.
Resource Optimization: Weather forecasts help optimize resource allocation during extreme weather events. Healthcare AI can learn to anticipate surges in demand for specific services (e.g., heatstroke during heatwaves) and optimise resource allocation accordingly.
By incorporating the strengths of weather AI, healthcare AI can become even more powerful in predicting, preventing, and managing health issues, leading to a more personalised and proactive healthcare system.
Mergers, Acquisitions, Growth and Strategy for Healthcare Technology companies
HealthTech M&A - Buy Side, Sell Side, Growth & Strategy services for companies in Europe, Middle East and Africa. Visit www.nelsonadvisors.co.uk
HealthTech M&A Newsletter from Nelson Advisors - HealthTech, Health IT, Digital Health Insights and Analysis. Subscribe Today! https://lnkd.in/e5hTp_xb
Healthcare Technology Buy Side, Sell Side, Growth & Strategy services for Founders, Owners and Investors. Email lloyd@nelsonadvisors.co.uk
Healthcare Technology Thought Leadership from Nelson Advisors – Market Insights, Analysis & Predictions. Visit https://lnkd.in/ezyUh5i
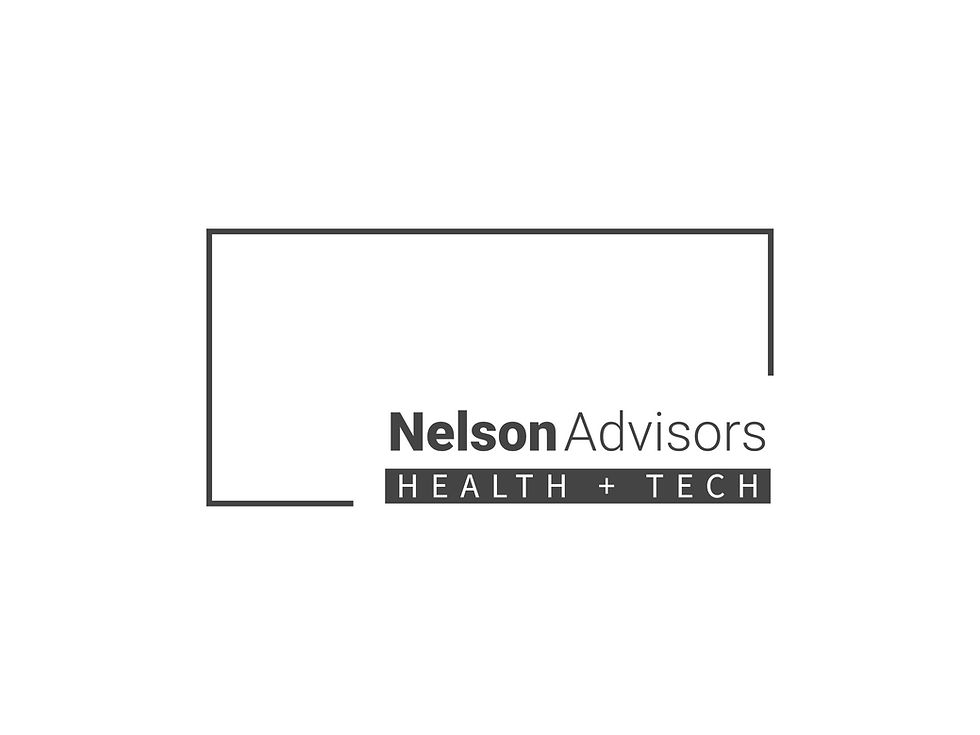
History of using machine learning in the weather industry
The history of machine learning in the weather industry can be roughly divided into three phases:
Early Beginnings (1970s - 2010s):
1970s: The initial use of AI in weather forecasting involved simple statistical methods and early computer algorithms. These algorithms mainly assisted with calculations and simulations within traditional numerical weather prediction models.
1990s - 2010s: Machine learning techniques like K-Nearest Neighbors and Support Vector Machines started gaining traction. These were primarily used for specific tasks like localised precipitation forecasts.
Limitations: This period was primarily focused on exploring the potential of machine learning within existing forecasting frameworks. The computational power and data availability limited the widespread adoption of complex AI models.
The Rise of Deep Learning (2010s - Present):
2010s: Advancements in computational power and the rise of deep learning techniques like artificial neural networks significantly impacted the field.
2020s: This period witnessed a rapid acceleration in the development and implementation of AI-powered weather forecasting models. Companies like Google DeepMind, NVIDIA, and Huawei made significant contributions.
Key developments:
Deep learning models achieved accuracy comparable to traditional models and even surpassed them in specific areas like short-term forecasting.
New applications like probabilistic forecasts and extreme weather event prediction emerged.
Revolutionising Weather Forecasting (Present and Future):
Current state: As of 2023, the field is experiencing continuous advancements with new research and model developments happening every few months.
Focus areas:
Integrating more complex architectures like 3D Neural Networks for global weather prediction.
Utilising Generative Adversarial Networks for tasks like precipitation forecasting.
Refining existing models and algorithms for even greater accuracy and efficiency.
Future outlook: Machine learning is expected to become the dominant force in weather forecasting, leading to:
Highly accurate and personalised weather predictions.
Improved early warning systems for severe weather events.
Enhanced climate modeling and analysis capabilities.
Overall, the history of machine learning in weather forecasting shows a rapid transformation from simple computational assistance to becoming a revolutionary force driving the future of weather prediction with ever-increasing accuracy and capabilities.
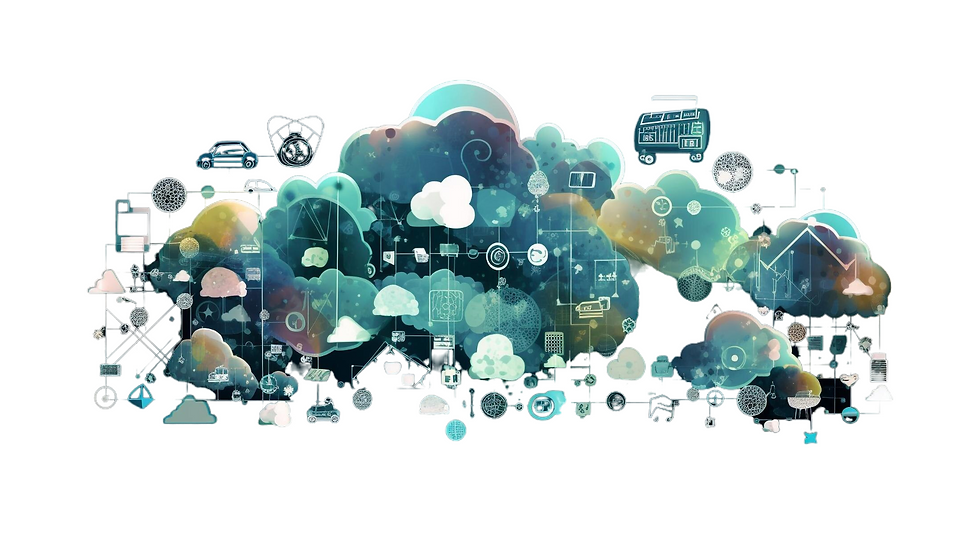
Healthcare AI can learn several valuable things from weather AI
Healthcare AI can indeed learn several valuable lessons from the advancements made in weather AI. Here's a summary of the key takeaways:
Data Integration and Analysis:
Handling Diverse Data: Weather AI excels at processing massive amounts of data from various sources like satellites, radars, and historical records. Healthcare AI can adopt similar techniques to integrate and analyse diverse patient data, including:
Medical records
Environmental factors
Genetic data
Sensor data from wearables
This allows for a more comprehensive understanding of individual health and the potential factors influencing it.
Advanced Modelling:
Identifying Complex Patterns: Weather AI utilises sophisticated algorithms to identify intricate relationships between various weather variables, leading to more accurate predictions. Healthcare AI can leverage similar techniques to analyse patient data and predict:
Disease risks
Potential complications
Individual health trends
This enables proactive interventions and personalised care plans.
Personalisation and Individualised Care:
Tailored Interventions: Weather forecasts are personalised based on location and individual needs. Healthcare AI can learn to create:
Personalised treatment plans
Medication schedules
Preventative measures based on a patient's specific health profile and environmental factors
This leads to more effective and targeted healthcare solutions.
Real-time Monitoring and Early Warning:
Dynamic Updates: Weather AI constantly updates forecasts based on real-time data. Healthcare AI can implement similar real-time monitoring systems to track:
Patient vital signs
Medication adherence
Potential risks
This enables early detection of potential health issues and timely intervention.
Additional Considerations:
Scalability: Weather AI models are designed to handle vast amounts of data across large geographical areas. This scalability can be valuable for healthcare AI in managing large patient populations and analysing data from geographically dispersed sources.
Constant Improvement: Weather AI is constantly evolving with advancements in machine learning algorithms and data processing capabilities. This continuous improvement should inspire healthcare AI to strive for ongoing development and refinement.
By applying these lessons, healthcare AI can significantly improve its ability to:
Analyse data: Extract insights from diverse and complex patient data.
Predict outcomes: Forecast disease risks, potential complications, and individual health trends.
Personalise care: Provide tailored treatment plans and preventative measures.
Enable early intervention: Monitor patients in real-time and identify potential risks early.
Ultimately, by learning from the successes of weather AI, healthcare AI can contribute to a more proactive, data-driven and personalised approach to healthcare, leading to improved patient outcomes and a healthier population.

Future of machine learning and deep learning in healthcare
The future of machine learning and deep learning in healthcare is brimming with exciting possibilities. Here are some key areas where we can expect significant advancements:
1. Personalised Medicine:
Precision diagnosis: Machine learning will analyse vast amounts of patient data, including medical history, genetic information, and lifestyle factors, to provide highly accurate personalised diagnoses and risk assessments for various diseases.
Tailored treatment plans: AI algorithms will suggest individualised treatment plans based on a patient's unique profile, potentially leading to more effective and targeted therapies.
Predictive analytics: AI models will predict potential health issues before they arise, enabling preventative measures and early intervention.
2. Enhanced Diagnostics and Imaging Analysis:
Deep learning algorithms will analyze medical images (X-rays, MRIs, etc.) with even greater accuracy than humans, leading to faster and more precise diagnoses. This could be crucial for early detection of cancers and other critical conditions.
AI-powered tools will assist surgeons during minimally invasive procedures, improving precision and reducing complications.
3. Drug Discovery and Development:
Machine learning can analyze vast datasets of molecular structures and patient data to accelerate drug discovery and development. This could lead to the creation of more effective and personalized medications with fewer side effects.
AI can optimise clinical trial design and patient selection, leading to faster and more efficient drug development processes.
4. Administrative Efficiency and Automation:
Machine learning can automate tedious tasks like claims processing, medical record management, and scheduling appointments, freeing up healthcare professionals to focus on patient care.
AI-powered chatbots can provide basic medical advice and answer patient questions, reducing the burden on healthcare systems.
5. Robotics and Surgical Assistance:
Robots assisted by AI will play an increasingly important role in surgery, performing complex procedures with greater precision and minimal invasiveness.
AI-powered exoskeletons can help patients with physical disabilities regain mobility and independence.
Additional Considerations:
Ethical considerations: As AI plays a more prominent role in healthcare, ethical concerns surrounding data privacy, bias in algorithms, and transparency in decision-making will need to be addressed carefully.
Regulatory landscape: Regulatory frameworks will need to adapt to ensure the safe and responsible use of AI in healthcare.
Overall, the future of machine learning and deep learning in healthcare is incredibly promising. These technologies have the potential to revolutionise healthcare by providing more accurate diagnoses, personalised treatments, and improved patient outcomes.
However, ethical considerations and regulatory frameworks need to evolve alongside these advancements to ensure responsible and beneficial implementation.
Mergers, Acquisitions, Growth and Strategy for Healthcare Technology companies
HealthTech M&A - Buy Side, Sell Side, Growth & Strategy services for companies in Europe, Middle East and Africa. Visit www.nelsonadvisors.co.uk
HealthTech M&A Newsletter from Nelson Advisors - HealthTech, Health IT, Digital Health Insights and Analysis. Subscribe Today! https://lnkd.in/e5hTp_xb
Healthcare Technology Buy Side, Sell Side, Growth & Strategy services for Founders, Owners and Investors. Email lloyd@nelsonadvisors.co.uk
Healthcare Technology Thought Leadership from Nelson Advisors – Market Insights, Analysis & Predictions. Visit https://lnkd.in/ezyUh5i
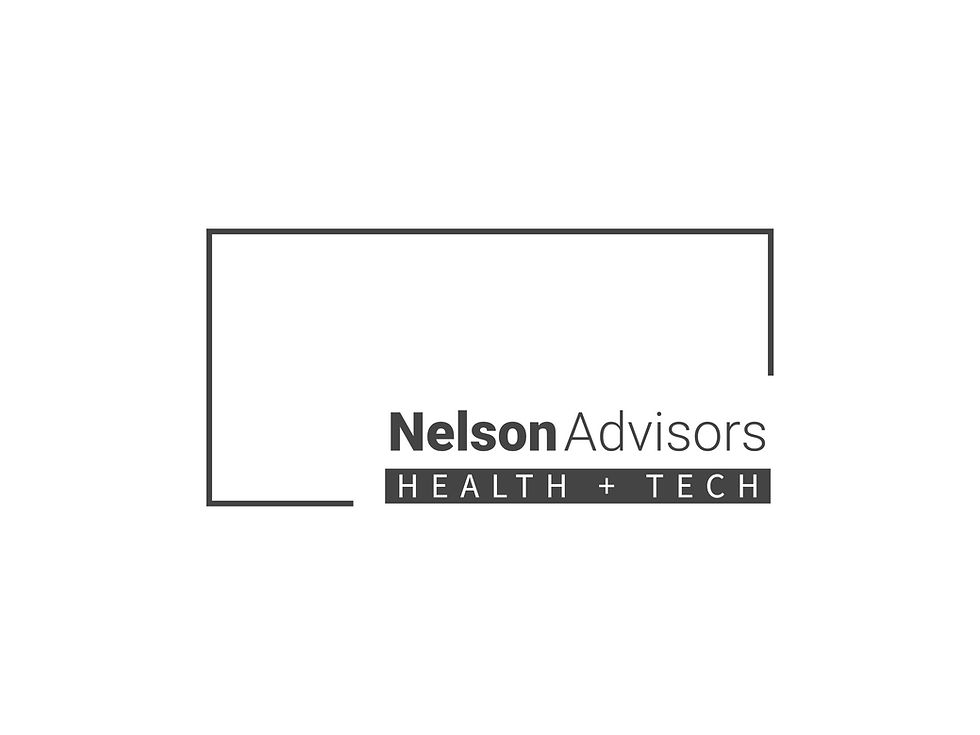
Comments