Hype v Hope: What is really going on with Healthcare AI across the NHS?
- Lloyd Price
- 10 minutes ago
- 7 min read
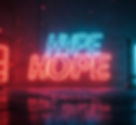
Beyond the Hype: What is really going on with Healthcare AI across the NHS?
Healthcare AI in the NHS is a mix of promising advancements and significant challenges, cutting through the buzz to focus on what’s actually happening. The NHS has been investing in AI to tackle real issues like early disease detection, operational efficiency and patient care improvement, but the rollout is far from seamless.
AI is being used to analyse massive datasets, think millions of patient records, to train models like Foresight, which predicts hospitalisations and conditions such as heart attacks. This model, developed with data from 57 million NHS patients, aims to flag risks early, potentially reducing emergency visits by identifying high risk individuals for proactive care.
For example, AI is helping predict frequent A&E users, allowing teams to offer targeted support and ease pressure on emergency services, with over 125 emergency departments using advanced AI and predictive analytics. There’s also practical progress in diagnostics: AI tools are speeding up cancer detection (eg. breast, lung, and bowel cancers) and stroke care, where decision support software has halved treatment times for stroke patients, tripling their chances of independent living post recovery. The NHS AI Lab has been central to these early successes, funding projects like the £21 million AI Diagnostic Fund announced in 2023 to roll out tools for faster diagnosis of cancers, strokes and heart conditions.
On the ground, AI is being integrated into specific areas like imaging and pathology, 34% of AI use in the NHS is in diagnostics, especially in acute and secondary care settings. For instance, AI driven imaging technology helps radiologists identify organs and growths in CT scans, whilst tools like Paige Prostate provide detailed cancer insights for more targeted treatments. Further afield, £32 million in funding is supporting 98 AI projects to improve prescription delivery and other efficiencies, highlighting a push toward practical, incremental gains rather than flashy overhauls.
But the reality isn’t all rosy. Scaling AI across the NHS is a struggle. Procurement processes are clunky, integration with existing systems is a nightmare and there’s a lack of alignment with what the NHS actually needs. Staff readiness is another hurdle, surveys show most NHS workers lack direct AI experience, though 57% are open to using it in their roles.
However, there’s a split: 44% see AI as an opportunity, while 43% worry it threatens jobs or professional status. Nurses and healthcare assistants are less enthusiastic than doctors, fearing a loss of human connection in care. Public sentiment mirrors this: while 54% of the public support AI for patient care, trust hinges on transparency and fairness.
Data privacy is a massive concern. The use of 57 million patient records to train models like Foresight has sparked ethical debates, with fears about data security and potential breaches. Critics argue the NHS’s data silos need to be unlocked for AI to work effectively, but this must be balanced with robust safeguards, something the NHS is still figuring out.
The Medicines and Healthcare products Regulatory Agency (MHRA) tightly regulates AI medical devices, but experts like Nell Thornton from the Health Foundation note that the sheer volume of AI models makes timely assessment tough. There’s also the risk of bias: early AI tools often failed to account for diverse populations, leading to unequal outcomes, though researchers are now addressing this.
Regulation and training are lagging. The NHS AI Lab and partnerships like the AI and Digital Regulations Service (launched with NICE, CQC, MHRA, and HRA) aim to guide safe adoption, but the rapid pace of AI development is outstripping regulatory capacity. Generative AI, for instance, is being explored for diagnostic accuracy and outcome forecasting, but it can “hallucinate” unreliable results, as Sir John Bell and others have warned. Staff need better training to handle these tools, some are even using models like ChatGPT for advice, which risks errors if not properly managed.
Financially, there’s early evidence of returns, AI diagnostics in non-elective care have saved an estimated £44 million across 150,000 patients, but the full impact will take years to materialise. The NHS is still in the early stages, with the government’s “three-seven” plan suggesting major reforms won’t hit until year four of a decade-long strategy. Meanwhile, the NHS App is being positioned as a Digital Care Hub to integrate AI tools, but past failures like the NHS Apps Library (shut down for poor quality and privacy issues) show the risks of rushing implementation.
In short, AI in the NHS is making real strides in diagnostics and efficiency, backed by significant funding and data resources. But it’s a messy, slow process plagued by integration issues, ethical concerns, and the need for better training and regulation. The hype promises transformation, but the reality is a cautious, uneven grind toward long term and meaningful change.

While the "hype" around AI in healthcare often focuses on futuristic, transformative changes, the reality across the NHS is a more nuanced picture of cautious progress, promising pilot projects, and significant challenges to widespread adoption.
Current State of AI in the NHS
Early Stages of Adoption: AI implementation is generally in its early stages, with many initiatives still in pilot phases rather than being fully integrated into routine care.
Focus on Specific Use Cases: Current applications tend to concentrate on areas like:
Diagnostics: Analysing medical images (X-rays, mammograms, brain scans) to support radiologists in making assessments, potentially speeding up diagnosis of conditions like cancer, stroke, and heart conditions.
Decision Support: Providing clinicians with tools to aid in treatment decisions. For example, AI is being used to predict patients at high risk of frequent A&E visits, enabling proactive intervention.
Administrative Efficiency: Automating tasks like appointment scheduling, staff rostering, and transcribing notes to free up staff time.
Remote Monitoring: Supporting virtual wards by analysing data from apps and wearable devices to monitor patients at home.
National Initiatives: NHS England has been actively exploring and funding AI projects through initiatives like the NHS AI Lab (which ran from 2019-2025) and the AI in Health and Care Award. These programs aimed to identify promising technologies and support their development and evaluation.
Learning and Evaluation: A key focus has been on learning from pilot projects, both successes and failures, to identify transferable lessons for wider AI implementation. There's an emphasis on evaluating the real-world impact of AI technologies on patient outcomes and efficiency.
Promising Examples and Projects
AI in Imaging: Tools analysing chest X-rays for lung cancer detection and retinal images for diabetic retinopathy are being explored and implemented.
Stroke Care: AI-powered decision support is being used to speed up stroke diagnosis, potentially tripling the chance of patients living independently after a stroke.
High Intensity Use (HIU) Services: AI is helping to identify patients at risk of frequent A&E attendance, allowing for targeted support and reducing hospital demand.
Virtual Wards: AI algorithms analyse data from wearable devices to remotely monitor patients, enabling them to receive care at home.
"Humphrey" Initiative: The government has launched a set of AI tools for civil servants, including those in the NHS, to streamline processes and improve efficiency in areas like consultation analysis and legal research.
Challenges to Widespread Implementation
Data and Digital Infrastructure: A lack of appropriate digital and data infrastructure is a major barrier to scaling AI solutions across the NHS. Integrating AI products requires robust systems.
Limited Awareness and Understanding: Some NHS leaders have limited familiarity with successful AI applications, particularly in non-clinical areas, leading to uncertainty about where to invest.
Financial Constraints: Procuring and implementing AI technologies can be expensive, posing a challenge for some NHS organisations.
Skills and Training: NHS staff require additional training and leadership support to effectively use and integrate AI into their workflows.
Integration with Existing Systems: Ensuring AI tools can seamlessly integrate with existing NHS digital systems is crucial but often complex.
Regulatory Clarity: Navigating the regulatory landscape for AI in healthcare, particularly in determining which applications are classified as medical devices, can be challenging.
Ethical Considerations and Bias: Ensuring AI algorithms are fair, unbiased, and do not exacerbate health inequalities is a significant concern. Issues around data privacy, transparency, and accountability also need careful consideration. For example, AI trained on predominantly white skin images may be less accurate in diagnosing conditions on black skin.
Maintaining Clinical Skills: Concerns exist about the potential for over-reliance on AI to deskill clinicians if not implemented thoughtfully.
Accountability and Responsibility: Determining who is accountable in case of AI errors or misdiagnosis is a complex legal and ethical issue.
The adoption of AI across the NHS is not a sudden revolution but rather a gradual evolution. While there are pockets of innovation and promising results from pilot projects, widespread adoption faces significant hurdles related to infrastructure, funding, skills, and ethical considerations. The focus is increasingly on learning from early experiences, developing ethical frameworks, and ensuring that AI implementation is driven by clear needs and delivers tangible benefits for both patients and staff. The emphasis is on augmenting human capabilities rather than replacing them entirely, with healthcare professionals retaining ultimate decision-making authority.
Nelson Advisors > Healthcare Technology M&A
.
Nelson Advisors specialise in mergers, acquisitions and partnerships for Digital Health, HealthTech, Health IT, Consumer HealthTech, Healthcare Cybersecurity, Healthcare AI companies based in the UK, Europe and North America. www.nelsonadvisors.co.uk
Nelson Advisors regularly publish Healthcare Technology thought leadership articles covering market insights, trends, analysis & predictions @ https://www.healthcare.digital
We share our views on the latest Healthcare Technology mergers, acquisitions and partnerships with insights, analysis and predictions in our LinkedIn Newsletter every week, subscribe today! https://lnkd.in/e5hTp_xb
Founders for Founders > We pride ourselves on our DNA as ‘HealthTech entrepreneurs advising HealthTech entrepreneurs.’ Nelson Advisors partner with entrepreneurs, boards and investors to maximise shareholder value and investment returns. www.nelsonadvisors.co.uk
#NelsonAdvisors #HealthTech #DigitalHealth #HealthIT #Cybersecurity #HealthcareAI #ConsumerHealthTech #Mergers #Acquisitions #Partnerships #Growth #Strategy #NHS #UK #Europe #USA #VentureCapital #PrivateEquity #Founders #BuySide #SellSide
Nelson Advisors LLP
Hale House, 76-78 Portland Place, Marylebone, London, W1B 1NT
Contact Us
Meet Us
Digital Health Rewired > 18-19th March 2025
NHS ConfedExpo > 11-12th June 2025
HLTH Europe > 16-19th June 2025
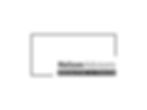