SNOMED CT: Healthcare AI's SuperPower
- Lloyd Price
- Apr 18
- 8 min read
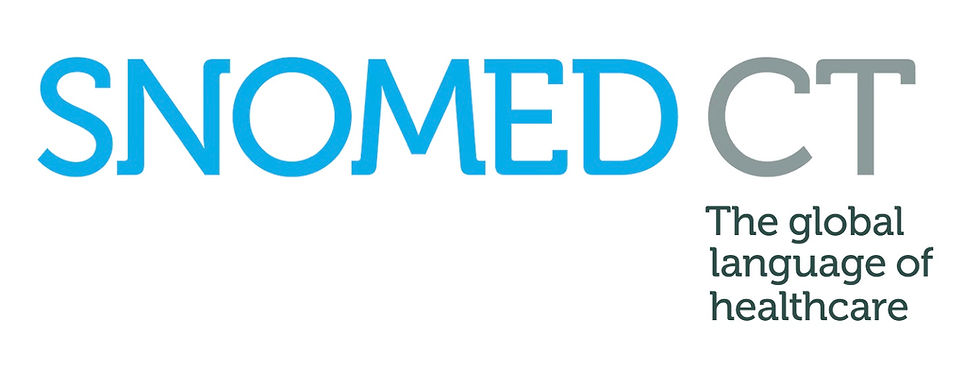
Exec Summary
SNOMED CT is the most comprehensive, multilingual clinical terminology system globally, with over 360,000 concepts, 1.5 million relationships, and 1 million descriptors (synonyms and definitions) covering diagnoses, procedures, symptoms, anatomy, and more.
Its structured, machine-readable format makes it ideal for AI applications, yet its complexity and implementation challenges often leave it underutilised compared to simpler standards like ICD-10.
SNOMED CT (Systematised Nomenclature of Medicine Clinical Terms) is a game-changer for AI-driven healthcare projects because it provides a standardised, interoperable framework for clinical data that enhances AI's ability to process, analyse, and derive insights from complex medical information
What is SNOMED CT?
SNOMED CT is the most comprehensive and precise multilingual clinical healthcare terminology in the world. It provides a standardised way to represent clinical phrases captured by clinicians in electronic health records.
SNOMED CT includes concepts, descriptions, and relationships, creating a rich, structured vocabulary covering diseases, symptoms, procedures, medications, and more. Its hierarchical structure and logical definitions allow for computer-understandable meanings and relationships between clinical concepts.
Why is SNOMED CT a superpower for AI?
SNOMED CT is a superpower for AI in healthcare because it transforms raw clinical data into a standardised, machine-readable and semantically rich format that supercharges AI’s capabilities.
Here’s why:
Universal Language for AI: SNOMED CT’s standardised clinical terminology eliminates inconsistencies across medical records, giving AI a clear, consistent "language" to process diagnoses, procedures, and symptoms, boosting accuracy in tasks like diagnostics or predictive modelling.
Data Integration at Scale: Its interoperable structure lets AI seamlessly combine data from EHRs, research databases, and global health systems, enabling large-scale, high-quality datasets for training robust models.
Semantic Superpowers: SNOMED’s hierarchical and relational ontology allows AI to "reason" by inferring connections (e.g., linking a symptom to a rare condition), enhancing capabilities in clinical decision support and personalised medicine.
Precision and Context: With granular, context-rich terms, SNOMED enables AI to capture nuanced clinical details, improving the precision of applications like risk stratification or treatment recommendations.
Global Reach: Adopted worldwide, SNOMED lets AI solutions scale across borders, supporting diverse populations and multinational research without data compatibility issues.
Real-Time Impact: By structuring data for instant AI processing, SNOMED powers real-time applications, from automated alerts in hospitals to population health monitoring.
In essence, SNOMED CT gives AI the ability to understand, integrate, and act on complex clinical data with unparalleled precision and scale, making it a cornerstone for transformative healthcare innovations.
While the potential of AI in healthcare is widely recognised, the foundational role that structured clinical terminologies like SNOMED CT can play in unlocking this potential is crucial and perhaps not always fully appreciated. As AI continues to evolve, leveraging the rich semantic information within SNOMED CT could be key to building more accurate, reliable, and impactful healthcare AI applications.
Nelson Advisors > HealthTech M&A
Nelson Advisors specialise in mergers, acquisitions and partnerships for Digital Health, HealthTech, Health IT, Healthcare Cybersecurity, Healthcare AI companies based in the UK, Europe and North America. www.nelsonadvisors.co.uk
We work with our clients to assess whether they should 'Build, Buy, Partner or Sell' in order to maximise shareholder value and investment returns. Email lloyd@nelsonadvisors.co.uk
Nelson Advisors regularly publish Healthcare Technology thought leadership articles covering market insights, trends, analysis & predictions @ https://www.healthcare.digital
We share our views on the latest Healthcare Technology mergers, acquisitions and partnerships with insights, analysis and predictions in our LinkedIn Newsletter every week, subscribe today! https://lnkd.in/e5hTp_xb
#HealthTech #DigitalHealth #HealthIT #NelsonAdvisors #Mergers #Acquisitions #Growth #Strategy #Cybersecurity #HealthcareAI #Partnerships #NHS #UK #Europe #USA #Canada
Nelson Advisors
Hale House, 76-78 Portland Place, Marylebone, London, W1B 1NT
Contact Us
Meet Us
Digital Health Rewired > 18-19th March 2025
NHS ConfedExpo > 11-12th June 2025
HLTH Europe > 16-19th June 2025
HIMSS AI in Healthcare > 10-11th July 2025
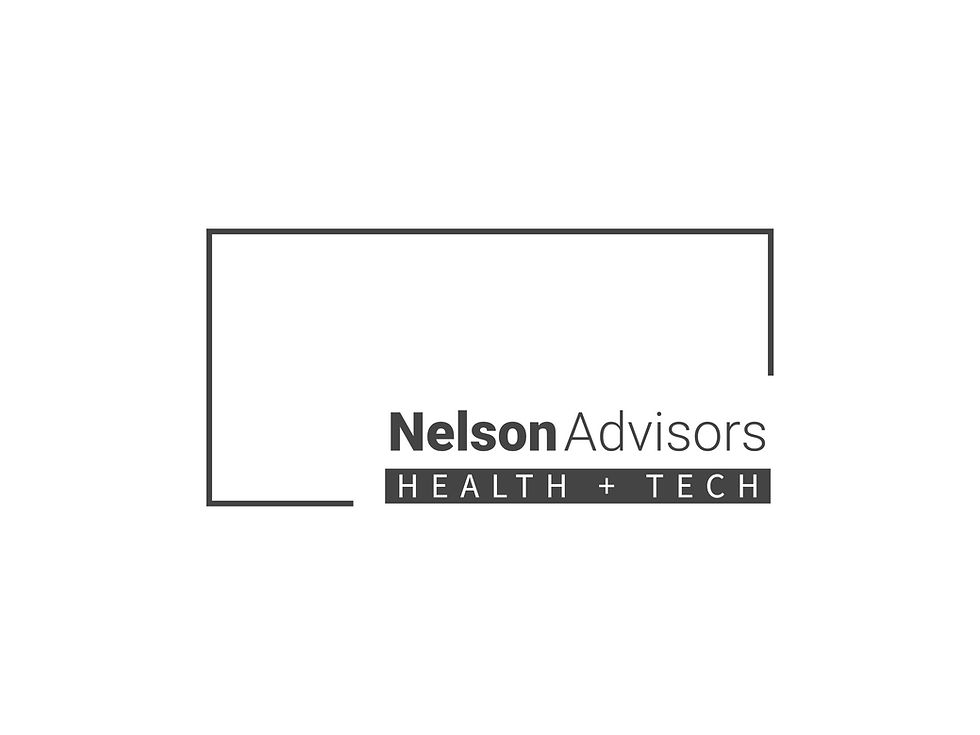
Features and Benefits of SNOMED CT
SNOMED CT (Systematised Nomenclature of Medicine Clinical Terms) is a critical enabler for AI in healthcare due to its robust features and the tangible benefits they deliver. Below is a concise breakdown of its key features and corresponding benefits for Healthcare AI:
Features of SNOMED CT
Comprehensive Clinical Terminology:
Covers over 350,000 concepts, including diagnoses, procedures, symptoms, medications, and more, with multilingual support.
Hierarchical Ontology:
Organises concepts in a structured, parent-child hierarchy (e.g., "pneumonia" under "respiratory infections").
Semantic Relationships:
Defines relationships between concepts (e.g., "caused by," "associated with"), enabling logical connections.
Machine-Readable Format:
Structured, coded data designed for computational processing.
Interoperability Standards:
Aligns with standards like HL7 FHIR, enabling integration across EHRs, registries, and health systems.
Global Adoption:
Used in over 80 countries, maintained by SNOMED International, ensuring consistency and scalability.
Regular Updates:
Continuously updated to reflect new medical knowledge, drugs, and procedures.
Extensibility:
Allows customisation with local extensions while maintaining global compatibility.
Benefits for Healthcare AI
Improved Data Quality and Consistency:
Standardised terms reduce ambiguity and errors in clinical data, providing clean, reliable datasets for training AI models, leading to higher accuracy in predictions and diagnostics.
Enhanced Interoperability:
Enables AI to integrate and analyse data from diverse sources (e.g., hospitals, labs, wearables), supporting comprehensive patient profiles and large-scale research.
Advanced Semantic Reasoning:
Hierarchical and relational structure allows AI to infer connections (e.g., linking symptoms to rare diseases), powering intelligent clinical decision support and personalised treatment plans.
Scalability Across Regions:
Global adoption ensures AI solutions can operate across borders, facilitating multinational studies and equitable healthcare innovations.
Real-Time Decision Support:
Machine-readable format enables AI to process SNOMED-coded data instantly, supporting applications like automated alerts, risk scoring, or treatment recommendations in clinical workflows.
Nuanced Insights:
Granular terminology captures detailed clinical context, allowing AI to perform precise tasks like cohort identification, disease progression modelling, or adverse event detection.
Adaptability to Evolving Needs:
Regular updates and extensibility ensure AI systems remain relevant as medical knowledge evolves, reducing obsolescence.
Population Health and Research:
Standardised data supports AI-driven epidemiology, trend analysis, and real-world evidence generation, accelerating drug discovery and public health interventions.
SNOMED CT’s structured, interoperable, and semantically rich framework empowers Healthcare AI to process complex clinical data with precision, scale, and adaptability. It drives better model performance, seamless data integration, and actionable insights, ultimately improving patient outcomes and advancing medical research.
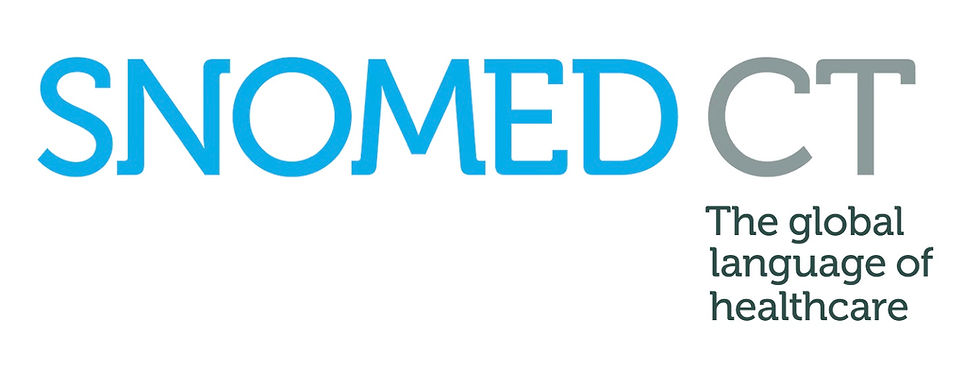
Five Examples of successful healthcare AI projects built on SNOMED coding
While specific, well-documented examples of AI-driven healthcare projects explicitly built on SNOMED CT (Systematized Nomenclature of Medicine Clinical Terms) coding are limited in the public domain due to the proprietary nature of many healthcare AI projects and the focus on broader interoperability standards, several initiatives and use cases leverage SNOMED CT’s standardized terminology to enhance AI applications.
Below are five examples of successful healthcare AI projects or initiatives that utilise SNOMED CT coding, drawn from available evidence and contextual understanding of its role in AI-driven healthcare.
1. Clinical Decision Support for Sepsis Detection (NHS England)
In England, NHS healthcare providers use SNOMED CT within electronic health record (EHR) systems to capture clinical terms, enabling AI-driven clinical decision support systems (CDSS) to detect early signs of sepsis. These systems analyse SNOMED CT-coded patient data (e.g., vital signs, symptoms, and lab results) to trigger real-time alerts for clinicians.
SNOMED CT provides a standardised vocabulary for encoding clinical findings (e.g., fever, tachycardia) and diagnoses, ensuring AI models can consistently interpret data across diverse EHR systems. Its hierarchical structure allows AI to identify patterns indicative of sepsis.
Improved early intervention for sepsis, reducing mortality rates and hospital stays. The standardized data enables AI to scale across NHS trusts, ensuring interoperability and consistent performance.
Source: NHS England’s SNOMED CT implementation for clinical systems.
2. IBM Watson Health Oncology Insights
IBM Watson Health uses AI to provide oncology decision support, analysing patient records to recommend personalised cancer treatments. While not exclusively built on SNOMED CT, Watson integrates SNOMED CT-coded data from EHRs to process clinical findings, diagnoses, and procedures.
SNOMED CT’s comprehensive terminology enables Watson to normalise unstructured clinical notes and free-text data into structured, machine-readable formats. Its semantic relationships support AI-driven reasoning, such as linking tumor characteristics to treatment protocols.
Enhanced precision in treatment recommendations, improved interoperability with hospital systems, and faster analysis of patient data for oncologists. The use of SNOMED CT ensures compatibility with global EHR standards.
Source: General knowledge of IBM Watson Health’s use of standardised terminologies like SNOMED CT, supported by SNOMED CT’s role in EHR interoperability.
3. Natural Language Processing (NLP) for Clinical Text Processing (SNOMED CT Free-Text Studies)
Research projects, such as those reviewed in the Journal of Medical Internet Research, have used SNOMED CT to process free-text clinical notes via NLP-driven AI systems. For example, AI models extract and encode clinical concepts (e.g., symptoms, diagnoses) from unstructured notes in EHRs, mapping them to SNOMED CT codes.
SNOMED CT serves as a reference terminology for data normalisation, enabling AI to convert free-text into structured data. Its synonyms and polyhierarchical structure allow NLP algorithms to handle variations in clinical language (e.g., “heart attack” vs. “myocardial infarction”).
Improved interoperability and secondary use of clinical data for research, quality reporting, and predictive analytics. One study demonstrated enhanced extraction of clinical events for cohort identification in chronic disease management.
Source: Journal of Medical Internet Research scoping review on SNOMED CT for free-text processing.
4. Allergy Management in Buenos Aires (SNOMED International Case Study)
A healthcare system in Buenos Aires implemented SNOMED CT to standardise allergy documentation, which was integrated into an AI-driven system to predict and manage allergic reactions. The AI analyzes SNOMED CT-coded allergy data alongside patient histories to flag potential risks during clinical encounters.
SNOMED CT’s detailed coding of allergens, reactions, and clinical contexts enables the AI to accurately identify and prioritise allergy-related risks. Its multilingual support ensures applicability in Spanish-speaking settings.
Reduced adverse events from allergic reactions, improved patient safety, and enhanced clinician decision-making through real-time risk alerts.
Source: SNOMED International case studies.
5. Breast Cancer Treatment Optimization in Sweden (SNOMED International Case Study)
In Sweden, SNOMED CT is used to standardise clinical data for breast cancer patients, which powers AI-driven analytics to optimise treatment plans. AI models analyze SNOMED CT-coded data (e.g., tumor staging, pathology results) to recommend evidence-based therapies.
SNOMED CT’s granular terminology ensures precise coding of cancer-related concepts, enabling AI to process complex datasets and identify treatment patterns. Its interoperability supports data sharing across Swedish healthcare facilities.
Improved treatment outcomes, reduced variability in care, and enhanced research capabilities through standardised data for population-level studies.
Source: SNOMED International case studies.
Challenges and Notes
Limited Public Documentation: Many AI projects using SNOMED CT are proprietary or not fully detailed in public literature, as noted in reviews of SNOMED CT use cases.
Complementary Standards: SNOMED CT is often used alongside other standards like LOINC or ICD-10, which complicates isolating its specific contribution to AI success.
Implementation Complexity: Mapping local terms to SNOMED CT and maintaining updates can be resource-intensive, but automated tools and NLP mitigate this.
These examples illustrate how SNOMED CT’s standardised, interoperable terminology enables AI to process clinical data accurately and at scale. From sepsis detection to radiology interoperability, SNOMED CT enhances AI’s ability to deliver real-time insights, improve patient outcomes, and support research
Nelson Advisors > HealthTech M&A
Nelson Advisors specialise in mergers, acquisitions and partnerships for Digital Health, HealthTech, Health IT, Healthcare Cybersecurity, Healthcare AI companies based in the UK, Europe and North America. www.nelsonadvisors.co.uk
We work with our clients to assess whether they should 'Build, Buy, Partner or Sell' in order to maximise shareholder value and investment returns. Email lloyd@nelsonadvisors.co.uk
Nelson Advisors regularly publish Healthcare Technology thought leadership articles covering market insights, trends, analysis & predictions @ https://www.healthcare.digital
We share our views on the latest Healthcare Technology mergers, acquisitions and partnerships with insights, analysis and predictions in our LinkedIn Newsletter every week, subscribe today! https://lnkd.in/e5hTp_xb
#HealthTech #DigitalHealth #HealthIT #NelsonAdvisors #Mergers #Acquisitions #Growth #Strategy #Cybersecurity #HealthcareAI #Partnerships #NHS #UK #Europe #USA #Canada
Nelson Advisors
Hale House, 76-78 Portland Place, Marylebone, London, W1B 1NT
Contact Us
Meet Us
Digital Health Rewired > 18-19th March 2025
NHS ConfedExpo > 11-12th June 2025
HLTH Europe > 16-19th June 2025
HIMSS AI in Healthcare > 10-11th July 2025
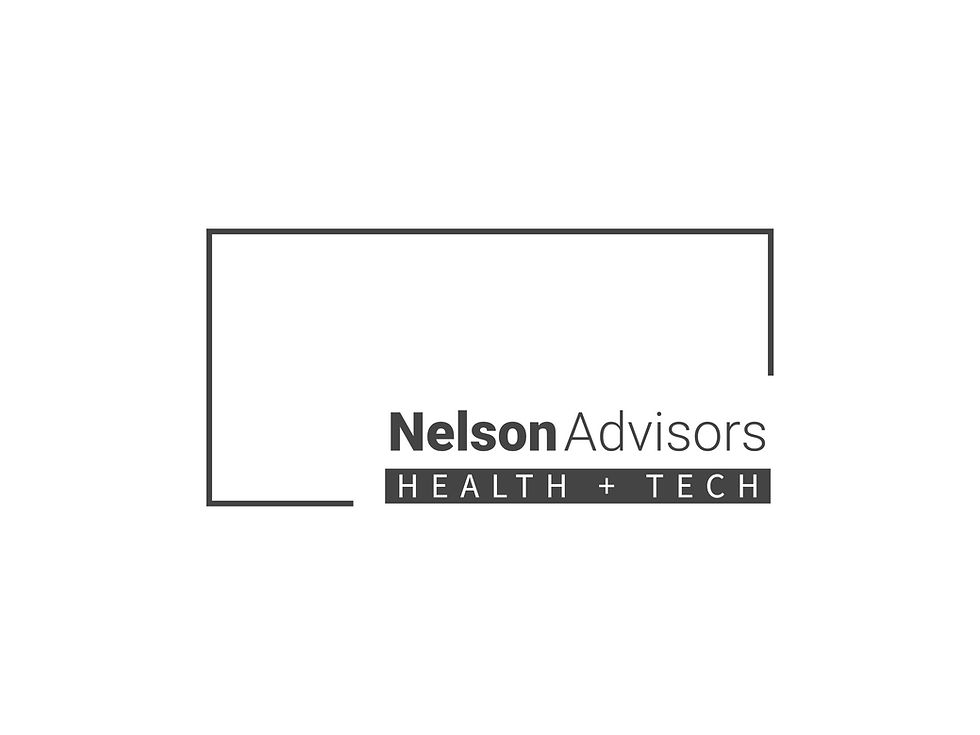
Commentaires